Quality control for AI
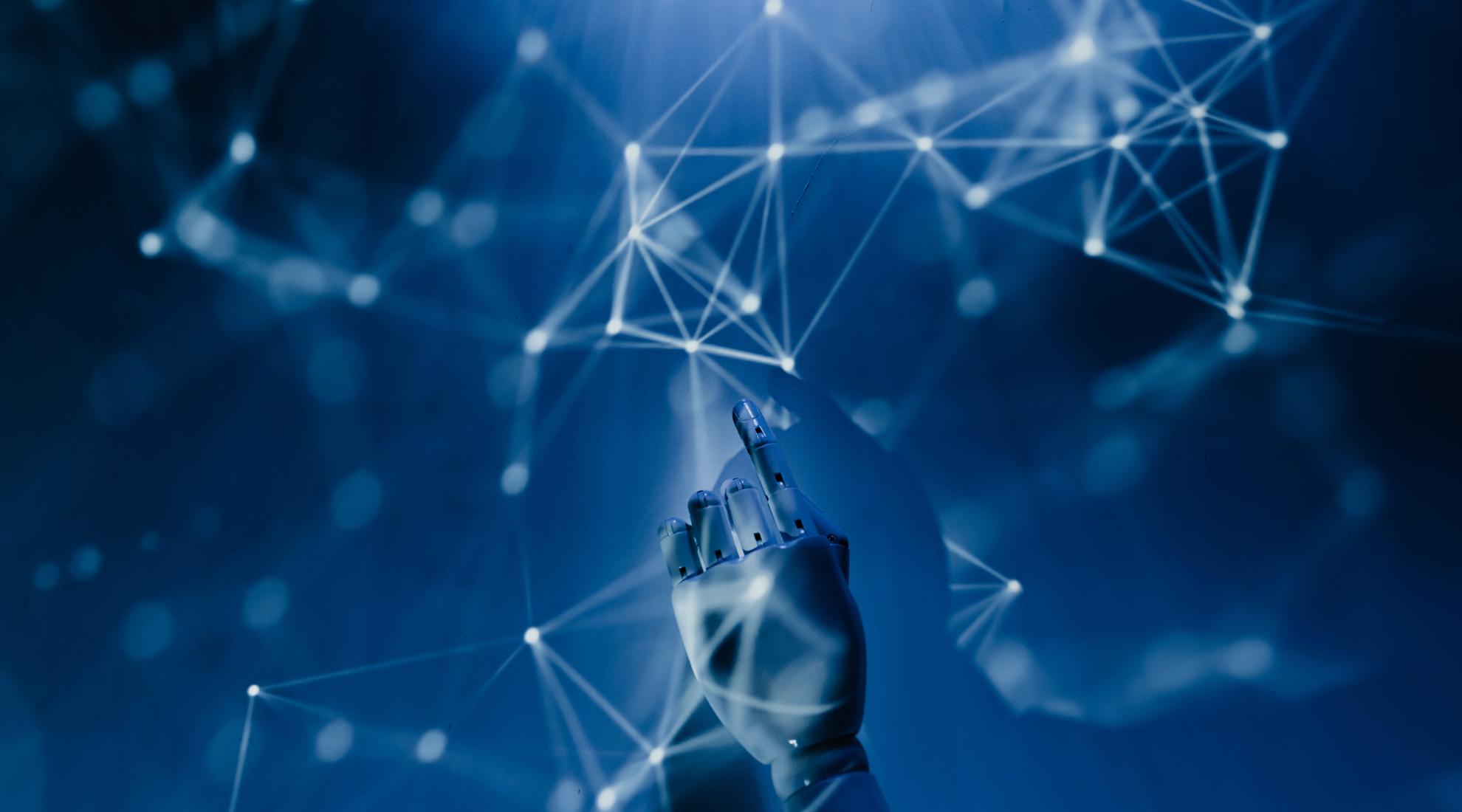
Artificial intelligence works best under laboratory conditions, but not always in practice. An ETH spin-off has developed a solution to make AI models more robust and reliable.
The artificial intelligence (AI) revolution, projected by PwC to contribute up to USD 15.7 trillion to the global economy in 2030 and more than the current output of China and India combined, faces one serious problem: machine learning models are not always robust and trustworthy. AI systems can make wrong decisions in production, resulting in misdiagnosed cancer patients, car accident fatalities, and more.
Automatic diagnosis to find blind spots in AI models
LatticeFlow, an ETH Zurich spin-off, has developed a platform that enables machine learning teams to automatically diagnose datasets and models, helping them find and fix critical model blind spots and data issues before the systems are migrated to their productive environments. The LatticeFlow concept and its first tools were initially developed in 2017 at ETH Zurich's Secure, Reliable, and Intelligent Systems Lab, where reliable, secure, robust and fair machine learning is part of the research focus. The lab's work brought two other successful spin-offs to the fore before LatticeFlow was founded in 2020. Discussions with over 100 companies about the difficulties of AI reliability led to the decision to scale up and automate the platform for auto-diagnosing data and AI models.
AI models developed for a very specific task may work in a limited test environment, but the real world is much more complex.
According to Petar Tsankov, co-founder and CEO of LatticeFlow, AI teams often still examine their models by hand - in the hope of finding systematic errors. This is an almost impossible undertaking, as the data sets are often enormous. LatticeFlow therefore wants to help them automate this process.
Reliable and robust automatic rail inspections
LatticeFlow already has a number of customers who are trialling its platform, including Swiss Federal Railways (SBB), which is using it to build a tool for automatic rail inspections. Specially dedicated trains equipped with cameras will collect high-quality image data of rail tracks which will then be processed by AI to identify any rail defects. Before the SBB hands off the inspection task to an AI system's 'eyes', it needs to ensure that the trained AI models correctly identify rail defects under different environmental conditions such rain or snow. The SBB has therefore partnered with LatticeFlow to assess the reliability of their models and to improve them if necessary.
The ETH spin-off recently won the Swiss AI Award 2022 in a competition with around 170 Swiss AI companies.